Understanding Labeled Image Datasets in Business
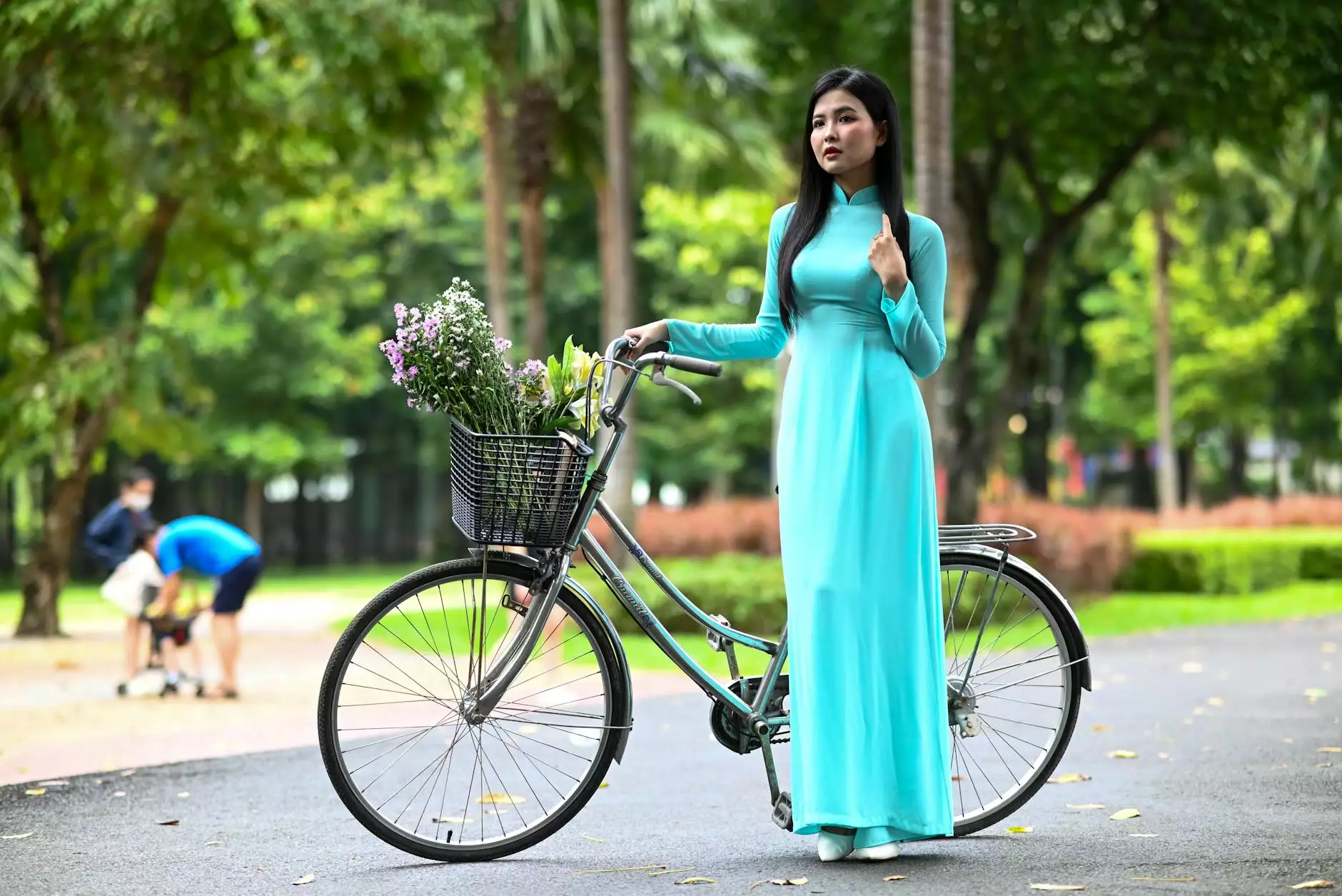
In the contemporary landscape of technology and business, the importance of data cannot be overstated. As organizations strive to leverage the power of artificial intelligence (AI) and machine learning (ML), one essential component that arises consistently is the utilization of labeled image datasets. This article delves deep into what labeled image datasets are, their relevance, and how businesses can effectively use them to drive innovation and efficiency.
What Are Labeled Image Datasets?
Labeled image datasets are collections of images that have been annotated or tagged with relevant information. Each image is associated with particular labels or classifications that help in training computer vision models. For instance, an image dataset used for distinguishing between cats and dogs would have images labeled as "cat" or "dog." The annotations facilitate the learning process of algorithms, enabling them to recognize patterns and make predictions based on visual inputs.
Why Are Labeled Image Datasets Crucial for Businesses?
Businesses today are increasingly relying on AI technologies to automate tasks, enhance customer experiences, and make data-driven decisions. Here are several key reasons why labeled image datasets play a pivotal role in this transformation:
- Enhancing Machine Learning Model Accuracy: Labeled datasets enable machine learning models to learn from training data, significantly improving their accuracy when making predictions.
- Fostering Innovation: By employing labeled image datasets, businesses can develop innovative solutions, from image recognition software to automated quality control systems.
- Optimizing Resource Allocation: Accurate predictions based on data analysis can help businesses optimize their resource allocation, ensuring maximum efficiency and cost-effectiveness.
- Improving Customer Insights: Through the analysis of labeled datasets, companies can garner insights into customer preferences and behavior, leading to tailored marketing strategies and improved product offerings.
Key Applications of Labeled Image Datasets in Various Industries
Different industries leverage labeled image datasets in unique ways. Here are some prominent applications:
1. Healthcare
In the healthcare sector, labeled image datasets are instrumental in developing diagnostic tools. For example, radiology relies heavily on image recognition capabilities for detecting abnormalities in X-rays and MRIs.
- Medical Imaging: Annotated datasets help train models to identify tumors and other pathologies.
- Predictive Analytics: Patient data, including images, can be analyzed to predict health outcomes and inform treatment plans.
2. Retail
Retailers use labeled datasets for improving user experience and inventory management.
- Visual Search Technology: Labeled image datasets enable visual search, allowing customers to find products by uploading images.
- Inventory Monitoring: Computer vision systems can identify products on shelves and alert staff when stock is low.
3. Automotive
The automotive industry utilizes labeled image datasets for developing self-driving technology.
- Object Recognition: Labeled datasets help vehicles recognize pedestrians, traffic signals, and road signs.
- Safety Features: Advanced Driver-Assistance Systems (ADAS) use image recognition to improve driver safety.
4. Agriculture
In agriculture, labeled image datasets facilitate crop management and monitoring.
- Pest Detection: Drones can capture images of fields, which are then analyzed for signs of pests and diseases.
- Yield Prediction: Image data can help predict crop yield based on growth patterns.
Choosing the Right Data Annotation Tool and Platform
Selecting the appropriate data annotation tool and platform is crucial for businesses aiming to leverage labeled image datasets effectively. Here are a few considerations:
1. Types of Annotations Offered
Different projects require different types of annotations, such as bounding boxes, polygons, or semantic segmentation. Ensure the tool you choose supports the types of annotations your project needs.
2. Scalability
Your chosen platform should be able to handle large volumes of images as your dataset grows. Look for platforms that offer scalability without compromising the quality of annotations.
3. Ease of Use
A user-friendly interface can significantly enhance productivity. Ensure the tool is intuitive and requires minimal training.
4. Collaboration Features
In many cases, team collaboration is essential for annotation projects. Choose tools that facilitate easy communication and task management among team members.
5. Cost-Effectiveness
Budget constraints are always a consideration. Compare different platforms and tools, ensuring you balance cost with the features offered.
Best Practices for Creating Labeled Image Datasets
To maximize the effectiveness of your labeled image datasets, follow these best practices:
1. Define Clear Annotation Guidelines
Establishing clear and concise guidelines ensures consistency in the labeling process, which is crucial for model performance.
2. Ensure Diversity in Datasets
Diverse datasets improve the generalizability of models. Ensure your datasets include various conditions, angles, and environments.
3. Utilize Quality Control
Implementing a quality control process helps catch labeling errors and inconsistencies, which can degrade model performance.
4. Continuously Update Datasets
As technology evolves and new data becomes available, regularly updating your labeled datasets is essential for maintaining the accuracy of AI models.
The Future of Labeled Image Datasets in Business
As AI technologies continue to advance, the role of labeled image datasets will only become more pronounced. With the advent of deep learning and neural networks, businesses can expect:
- Increased Demand: More industries will seek labeled datasets to develop more sophisticated AI applications.
- Automation in Annotation: Emerging tools may automate aspects of the annotation process, reducing time and labor costs.
- Integration with Other Data Types: Combining labeled image data with other data types like text and audio will yield more robust AI systems.
Conclusion
In summary, labeled image datasets have emerged as a foundational element in the success of AI and ML applications across various industries. By understanding their significance, properly selecting data annotation tools and platforms, and implementing best practices, businesses can unlock new potentials that drive efficiency and innovation. As the landscape of technology evolves, so will the methodologies surrounding the creation and utilization of labeled image datasets, making it essential for forward-thinking organizations to stay informed and adapt.
Keylabs.ai stands at the forefront of providing top-notch data annotation tools and data annotation platforms that empower businesses to harness the full potential of their labeled datasets. Embrace the future of business intelligence with the right tools and strategies for success.